Nicolas Papernot
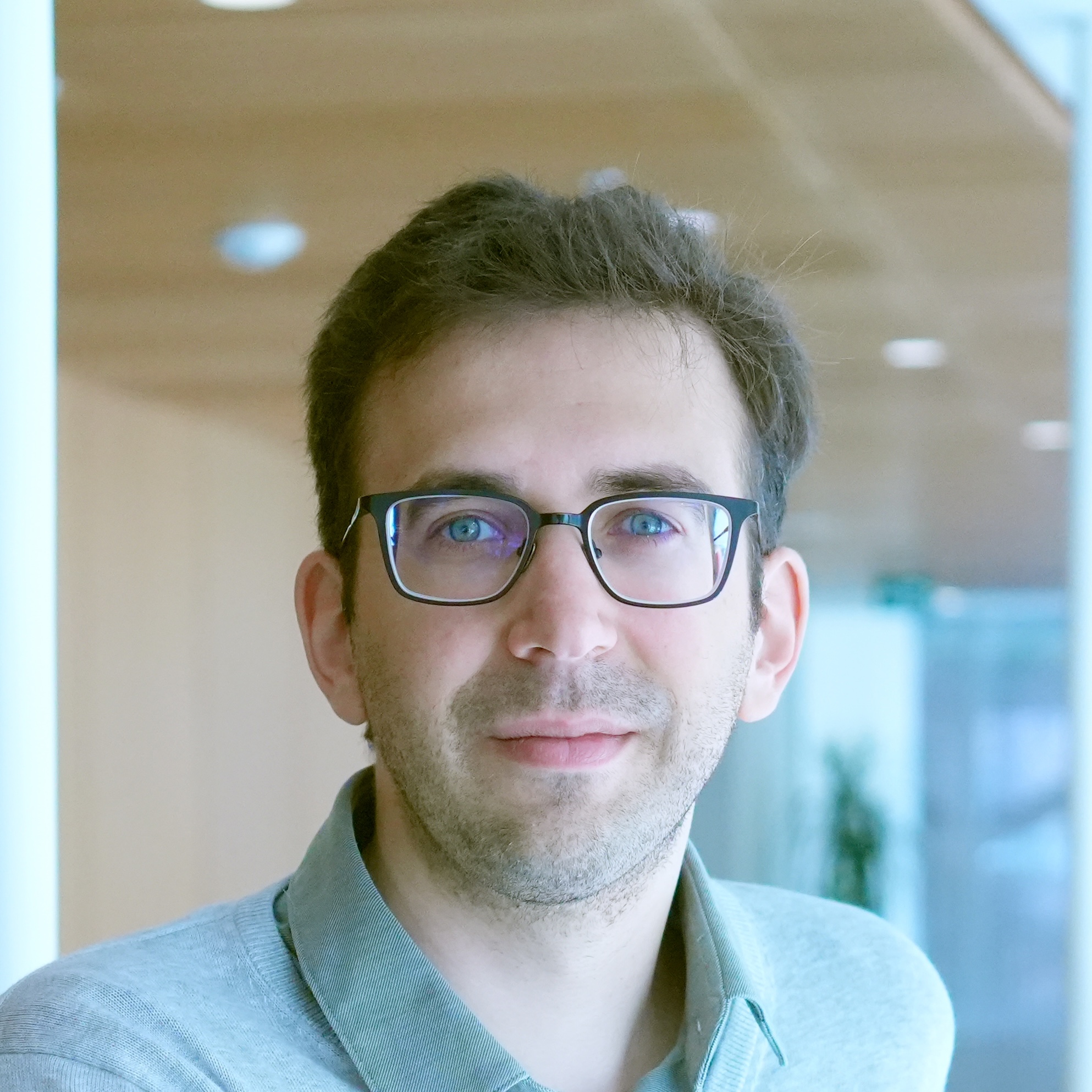
I am an Assistant Professor at the University of Toronto, in the Department of Electrical and Computer Engineering, the Department of Computer Science, and the Faculty of Law. I am also a faculty member at the Vector Institute where I hold a Canada CIFAR AI Chair, and a faculty affiliate at the Schwartz Reisman Institute.
My research interests are at the intersection of security, privacy, and machine learning. If you would like to learn more about my research, I recommend reading the blog posts I co-authored on cleverhans.io, for example about proof-of-learning, collaborative learning beyond federation, dataset inference, machine unlearning, differentially private ML, or adversarial examples.
I was named an Alfred P. Sloan Research Fellow in Computer Science in 2022, a Member of the Royal Society of Canada College in 2023, an AI2050 Early Career Fellow By Schmidt Sciences in 2024, and received the McCharles Prize for Early Career Research Distinction in 2024.
My research has been cited in the press, including the BBC, New York Times, Popular Science, The Atlantic, the Wall Street Journal and Wired. I co-founded and served as a Program Committee Co-Chair of the IEEE Conference on Secure and Trustworthy Machine Learning (SaTML) in 2023 and 2024. I earned my Ph.D. in Computer Science and Engineering at the Pennsylvania State University, working with Prof. Patrick McDaniel and supported by a Google PhD Fellowship. Upon graduating, I joined Google Brain for a year; I continue to spend time at Google DeepMind.
Email: [email protected]
Office: Pratt 484E and SRIC (the Vector Institute lobby is on the 11th floor)
Mail/Packages: 10 King's College Road, Room SFB540, Toronto, ON M5S 3G4, Canada
Recent & selected older publications
A complete list of publications is available in my CV.
- 2024
- A False Sense of Safety: Unsafe Information Leakage in Safe AI Responses. David Glukhov, Ziwen Han, Ilia Shumailov, Vardan Papyan, Nicolas Papernot. preprint
- Gradients Look Alike: Sensitivity is Often Overestimated in DP-SGD. Anvith Thudi, Hengrui Jia, Casey Meehan, Ilia Shumailov, Nicolas Papernot. Proceedings of the 33rd USENIX Security Symposium. conference
- Auditing Private Prediction. Karan Chadha, Matthew Jagielski, Nicolas Papernot, Christopher A. Choquette-Choo, Milad Nasr. Proceedings of the 41st International Conference on Machine Learning, Vienna, Austria. conference
- The Fundamental Limits of Least-Privilege Learning. Theresa Stadler, Bogdan Kulynych, Michael Gastpar, Nicolas Papernot, Carmela Troncoso. Proceedings of the 41st International Conference on Machine Learning, Vienna, Austria. conference
- Position Paper: Rethinking LLM Censorship as a Security Problem. David Glukhov, Ilia Shumailov, Yarin Gal, Nicolas Papernot, Vardan Papyan. Proceedings of the 41st International Conference on Machine Learning, Vienna, Austria. conference
- Fairness Feedback Loops: Training on Synthetic Data Amplifies Bias. Sierra Calanda Wyllie, Ilia Shumailov, Nicolas Papernot. Proceedings of the 2024 ACM Conference on Fairness, Accountability, and Transparency. conference
- From Differential Privacy to Bounds on Membership Inference: Less can be More. Anvith Thudi, Ilia Shumailov, Franziska Boenisch, Nicolas Papernot. Transactions on Machine Learning Research. journal
- Unlearnable Algorithms for In-context Learning. Andrei Ioan Muresanu, Anvith Thudi, Michael R. Zhang, Nicolas Papernot. preprint
- Decentralised, Collaborative, and Privacy-preserving Machine Learning for Multi-Hospital Data. Congyu Fang, Adam Dziedzic, Lin Zhang, Laura Oliva, Amol Verma, Fahad Razak, Nicolas Papernot, Bo Wang. eBioMedicine Volume 101. journal
- Memorization in Self-Supervised Learning Improves Downstream Generalization. Wenhao Wang, Muhammad Ahmad Kaleem, Adam Dziedzic, Michael Backes, Nicolas Papernot, Franziska Boenisch. Proceedings of the 12th International Conference on Learning Representations. conference
- Confidential-DPproof: Confidential Proof of Differentially Private Training. Ali Shahin Shamsabadi, Gefei Tan, Tudor Ioan Cebere, Aurélien Bellet, Hamed Haddadi, Nicolas Papernot, Xiao Wang, Adrian Weller. Proceedings of the 12th International Conference on Learning Representations. conference (+spotlight)
- Exploring Strategies for Guiding Symbolic Analysis with Machine Learning Prediction. Mingyue Yang, David Lie, Nicolas Papernot. 31st IEEE International Conference on Software Analysis, Evolution and Reengineering. conference
- 2023
- The Curse of Recursion: Training on Generated Data Makes Models Forget. Ilia Shumailov, Zakhar Shumaylov, Yiren Zhao, Yarin Gal, Nicolas Papernot, Ross Anderson. preprint
- Robust and Actively Secure Serverless Collaborative Learning. Olive Franzese, Adam Dziedzic, Christopher A. Choquette-Choo, Mark R. Thomas, Muhammad Ahmad Kaleem, Stephan Rabanser, Congyu Fang, Somesh Jha, Nicolas Papernot, Xiao Wang. Proceedings of the 37th Conference on Neural Information Processing Systems. conference
- Regulation Games for Trustworthy Machine Learning. Mohammad Yaghini, Patty Liu, Franziska Boenisch, Nicolas Papernot. NeurIPS 2023 Workshop on Regulatable ML. workshop
- Training Private Models That Know What They Don’t Know. Stephan Rabanser, Anvith Thudi, Abhradeep Thakurta, Krishnamurthy Dvijotham, Nicolas Papernot. Proceedings of the 37th Conference on Neural Information Processing Systems. conference
- Have it your way: Individualized Privacy Assignment for DP-SGD. Franziska Boenisch, Christopher Mühl, Adam Dziedzic, Roy Rinberg, Nicolas Papernot. Proceedings of the 37th Conference on Neural Information Processing Systems. conference
- Flocks of Stochastic Parrots: Differentially Private Prompt Learning for Large Language Models. Haonan Duan, Adam Dziedzic, Nicolas Papernot, Franziska Boenisch. Proceedings of the 37th Conference on Neural Information Processing Systems. conference
- Proof-of-Learning is Currently More Broken Than You Think. Congyu Fang, Hengrui Jia, Anvith Thudi, Mohammad Yaghini, Christopher A. Choquette-Choo, Natalie Dullerud, Varun Chandrasekaran, Nicolas Papernot. Proceedings of the 8th IEEE European Symposium on Security and Privacy, Delft, Netherlands. conference
- Reconstructing Individual Data Points in Federated Learning Hardened with Differential Privacy and Secure Aggregation. Franziska Boenisch, Adam Dziedzic, Roei Schuster, Ali Shahin Shamsabadi, Ilia Shumailov, Nicolas Papernot. Proceedings of the 8th IEEE European Symposium on Security and Privacy, Delft, Netherlands. conference
- When the Curious Abandon Honesty: Federated Learning Is Not Private. Franziska Boenisch, Adam Dziedzic, Roei Schuster, Ali Shahin Shamsabadi, Ilia Shumailov, Nicolas Papernot. Proceedings of the 8th IEEE European Symposium on Security and Privacy, Delft, Netherlands. conference
- Losing Less: A Loss for Differentially Private Deep Learning. Ali Shahin Shamsabadi, Nicolas Papernot. Proceedings on Privacy Enhancing Technologies, Lausanne, Switzerland. conference
- Architectural Backdoors in Neural Networks. Mikel Bober-Irizar, Ilia Shumailov, Yiren Zhao, Robert Mullins, Nicolas Papernot. Proceedings of the 2023 IEEE/CVF Conference on Computer Vision and Pattern Recognition, Vancouver, Canada. conference
- Measuring Forgetting of Memorized Training Examples. Matthew Jagielski, Om Thakkar, Florian Tramer, Daphne Ippolito, Katherine Lee, Nicholas Carlini, Eric Wallace, Shuang Song, Abhradeep Guha Thakurta, Nicolas Papernot, Chiyuan Zhang. Proceedings of the 11th International Conference on Learning Representations. conference
- Confidential-PROFITT: Confidential PROof of FaIr Training of Trees. Ali Shahin Shamsabadi, Sierra Calanda Wyllie, Nicholas Franzese, Natalie Dullerud, Sébastien Gambs, Nicolas Papernot, Xiao Wang, Adrian Weller. Proceedings of the 11th International Conference on Learning Representations. conference (+oral)
- Private Multi-Winner Voting for Machine Learning. Adam Dziedzic, Christopher A. Choquette-Choo, Natalie Dullerud, Vinith Menon Suriyakumar, Ali Shahin Shamsabadi, Muhammad Ahmad Kaleem, Somesh Jha, Nicolas Papernot, Xiao Wang. Proceedings on Privacy Enhancing Technologies, Lausanne, Switzerland. conference
- Differentially Private Speaker Anonymization. Ali Shahin Shamsabadi, Brij Mohan Lal Srivastava, Aurelien Bellet, Nathalie Vauquier, Emmanuel Vincent, Mohamed Maouche, Marc Tommasi, Nicolas Papernot. Proceedings on Privacy Enhancing Technologies, Lausanne, Switzerland. conference
- Tubes Among Us: Analog Attack on Automatic Speaker Identification. Shimaa Ahmed, Yash Wani, Ali Shahin Shamsabadi, Mohammad Yaghini, Ilia Shumailov, Nicolas Papernot, Kassem Fawaz. Proceedings of the 32nd USENIX Security Symposium. conference
- 2022 & earlier
- Washing The Unwashable: On The (Im)possibility of Fairwashing Detection. Ali Shahin Shamsabadi, Mohammad Yaghini, Natalie Dullerud, Sierra Wyllie, Ulrich Aïvodji, Aisha Alaagib Alryeh Mkean, Sébastien Gambs, Nicolas Papernot. Proceedings of the 36th Conference on Neural Information Processing Systems. conference
- On the Limitations of Stochastic Pre-processing Defenses. Yue Gao, Ilia Shumailov, Kassem Fawaz, Nicolas Papernot. Proceedings of the 36th Conference on Neural Information Processing Systems. conference
- Selective Classification Via Neural Network Training Dynamics. Stephan Rabanser, Anvith Thudi, Kimia Hamidieh, Adam Dziedzic, Nicolas Papernot. preprint
- On the Necessity of Auditable Algorithmic Definitions for Machine Unlearning. Anvith Thudi, Hengrui Jia, Ilia Shumailov, Nicolas Papernot. Proceedings of the 31st USENIX Security Symposium. conference
- Increasing the Cost of Model Extraction with Calibrated Proof of Work. Adam Dziedzic, Muhammad Ahmad Kaleem, Yu Shen Lu, Nicolas Papernot. Proceedings of the 10th International Conference on Learning Representations. conference (+spotlight)
- A Zest of LIME: Towards Architecture-Independent Model Distances. Hengrui Jia, Hongyu Chen, Jonas Guan, Ali Shahin Shamsabadi, Nicolas Papernot. Proceedings of the 10th International Conference on Learning Representations. conference
- Hyperparameter Tuning with Renyi Differential Privacy. Nicolas Papernot, Thomas Steinke. Proceedings of the 10th International Conference on Learning Representations. conference (+outstanding paper award)
- Bad Character Injection: Imperceptible Attacks on NLP Models. Nicholas Boucher, Ilia Shumailov, Ross Anderson, Nicolas Papernot. Proceedings of the 43rd IEEE Symposium on Security and Privacy, San Francisco, CA. conference
- Towards More Robust Keyword Spotting for Voice Assistants. Shimaa Ahmed, Ilia Shumailov, Nicolas Papernot, Kassem Fawaz. Proceedings of the 31st USENIX Security Symposium. conference
- Manipulating SGD with Data Ordering Attacks. Ilia Shumailov, Zakhar Shumaylov, Dmitry Kazhdan, Yiren Zhao, Nicolas Papernot, Murat A. Erdogdu, Ross Anderson. Proceedings of the 35th Conference on Neural Information Processing Systems. conference
- Proof-of-Learning: Definitions and Practice. Hengrui Jia, Mohammad Yaghini, Christopher A. Choquette-Choo, Natalie Dullerud, Anvith Thudi, Varun Chandrasekaran, Nicolas Papernot. Proceedings of the 42nd IEEE Symposium on Security and Privacy, San Francisco, CA. conference
- Entangled Watermarks as a Defense against Model Extraction. Hengrui Jia, Christopher A. Choquette-Choo, Varun Chandrasekaran, Nicolas Papernot. Proceedings of the 30th USENIX Security Symposium. conference
- Sponge Examples: Energy-Latency Attacks on Neural Networks. Ilia Shumailov, Yiren Zhao, Daniel Bates, Nicolas Papernot, Robert Mullins, Ross Anderson. Proceedings of the 6th IEEE European Symposium on Security and Privacy, Vienna, Austria. conference
- CaPC Learning: Confidential and Private Collaborative Learning. Christopher A. Choquette-Choo, Natalie Dullerud, Adam Dziedzic, Yunxiang Zhang, Somesh Jha, Nicolas Papernot, Xiao Wang. Proceedings of the 9th International Conference on Learning Representations. conference
- Dataset Inference: Ownership Resolution in Machine Learning. Pratyush Maini, Mohammad Yaghini, Nicolas Papernot. Proceedings of the 9th International Conference on Learning Representations. conference (+spotlight)
- Machine Unlearning. Lucas Bourtoule, Varun Chandrasekaran, Christopher A. Choquette-Choo, Hengrui Jia, Adelin Travers, Baiwu Zhang, David Lie, Nicolas Papernot. Proceedings of the 42nd IEEE Symposium on Security and Privacy, San Francisco, CA. conference
- Analyzing and Improving Representations with the Soft Nearest Neighbor Loss. Nicholas Frosst, Nicolas Papernot, Geoffrey Hinton. Proceedings of the 36th International Conference on Machine Learning, Long Beach, CA. conference
- Scalable Private Learning with PATE. Nicolas Papernot, Shuang Song, Ilya Mironov, Ananth Raghunathan, Kunal Talwar, Ulfar Erlingsson. Proceedings of the 6th International Conference on Learning Representations, Vancouver, Canada. conference
- Towards the Science of Security and Privacy in Machine Learning. Nicolas Papernot, Patrick McDaniel, Arunesh Sinha, and Michael Wellman. Proceedings of the 3rd IEEE European Symposium on Security and Privacy, London, UK. conference
- Semi-supervised Knowledge Transfer for Deep Learning from Private Training Data. Nicolas Papernot, Martin Abadi, Ulfar Erlingsson, Ian Goodfellow, and Kunal Talwar. Proceedings of the 5th International Conference on Learning Representations, Toulon, France. conference (+best paper)
- Practical Black-Box Attacks against Machine Learning. Nicolas Papernot, Patrick McDaniel, Ian Goodfellow, Somesh Jha, Z.Berkay Celik, and Ananthram Swami. Proceedings of the 2017 ACM Asia Conference on Computer and Communications Security, Abu Dhabi, UAE. conference
- Transferability in Machine Learning: from Phenomena to Black-Box Attacks using Adversarial Samples. Nicolas Papernot, Patrick McDaniel, and Ian Goodfellow. technical report
- The Limitations of Deep Learning in Adversarial Settings. Nicolas Papernot, Patrick McDaniel, Somesh Jha, Matt Fredrikson, Z. Berkay Celik, and Ananthram Swami. Proceedings of the 1st IEEE European Symposium on Security and Privacy, Saarbrucken, Germany. conference
Research group
Current students and postdocs
- Michael Menart: Postdoctoral Fellow (staring Fall 2024, co-advised with Aleksandar Nikolov)
- Tom Blanchard: MASc student (starting Fall 2024)
- Angéline Pouget: Visiting Masters student (Spring 2024 - Fall 2024)
- Andrew Magnuson: Engineering Science student (Started Winter 2024)
- David Glukhov: PhD student (started Winter 2023, co-advised with Vardan Papyan)
- Emmy Fang: PhD student (started Fall 2023, co-advised with Bo Wang) OGS Scholar, DiDi Award
- Pascale Gourdeau: Postdoctoral Fellow (started Fall 2023, co-advised with Shai Ben-David) NSERC Fellow
- Haonan Duan: PhD student (started Fall 2021, co-advised with Chris Maddison)
- Anvith Thudi: PhD student (started Fall 2022, co-advised with Chris Maddison) Vanier Scholar
- Sierra Wyllie: Engineering Science student (started Summer 2021)
- Mohammad Yaghini: PhD student (started Fall 2020) Meta PhD Fellow
- Stephan Rabanser: PhD student (started Fall 2020)
- Jonas Guan: PhD student (started Fall 2020)
- Jiaqi Wang: MASc student (started Fall 2020, co-advised with David Lie) OGS Scholar
- Nick Jia: PhD student (started Fall 2020) Vector Scholarship, Mary H. Beatty Fellow, OGS Scholar
- Mingyue Yang: PhD student (started Winter 2020, co-advised with David Lie)
Past students and postdocs
- Ziwen Han: Undergraduate student (Fall 2023 - Summer 2024) currently Research Engineer at Scale AI
- Tudor Cebere: Visiting PhD student (Fall 2023 - Winter 2024) currently PhD student at INRIA
- Andy Liu: Engineering Science student (Fall 2023 - Summer 2024) currently ASICs Engineer at Qualcomm
- Berivan Isik: Visiting PhD student (Summer 2023) currently PhD student at Stanford University
- Karan Chadha: Google Brain Intern (Summer 2023, co-hosted with Matthew Jagielski) currently Research Scientist at Meta
- Camille Bruckmann: Engineering Science student (Fall 2022 - Summer 2023) currently Software Engineer at Microsoft
- Si Cheng (Steven) Zhong: Engineering Science student (Fall 2022 - Summer 2023) currently MS student at University of Toronto
- Franziska Boenisch: Postdoctoral Fellow (Fall 2022 - Summer 2023) currently Assistant Professor at CISPA Helmholtz Center for Information Security
- Shimaa Ahmed: Visiting PhD student (Summer 2022) currently PhD student at University of Wisconsin-Madison
- Roy Rinberg: Research Intern (Summer 2022) currently PhD student at Harvard University
- Patty Liu: Engineering Science student (May 2022 - August 2023) currently PhD student at Princeton University
- Mark Thomas: Research Intern (Summer 2022) currently MS student at the University of Toronto
- Avital Shafran: Visiting PhD student (Summer 2022) currently PhD student at the Hebrew University of Jerusalem
- Thorsten Eisenhofer: Visiting PhD student (Summer 2022) currently Postdoctoral Fellow at TU Berlin
- Yannis Cattan: Research Intern (Summer 2022) currently Masters student at ENS Paris-Saclay (MVA)
- Roei Schuster: Postdoctoral Fellow (2021-2022) currently CTO at Context AI
- Ilia Shumailov: Postdoctoral Fellow (started Fall 2021, co-advised with Kassem Fawaz) currently Research Scientist at Google DeepMind and Junior Research Fellow at Oxford
- Aditi Misra: Engineering Science student (Fall 2021 - Spring 2024) currently Quantitative Researcher at Squarepoint
- Hongyu (Charlie) Chen: Engineering Science student (Fall 2021 - Summer 2022) currently Machine Learning Engineer at Cohere.ai
- Muhammad Ahmad Kaleem: Engineering Science student (Summer 2021 - Summer 2023) currently Engineering Science student at University of Toronto
- Aisha Alaagib: Research Intern (Summer 2021) currently Research Intern at MILA
- Armin Ale: Engineering Science student (Summer 2021 - Summer 2022) currently Software Engineer at Intel
- Ali Shahin Shamsabadi: Research Intern (Winter 2021 - Fall 2021) currently Privacy Researcher at Brave
- Adam Dziedzic: Postdoctoral Fellow (Fall 2020 - Summer 2023) currently Assistant Professor at CISPA Helmholtz Center for Information Security
- Natalie Dullerud: MS student (Fall 2020 - Summer 2022) currently PhD Student at Stanford
- Steven Xia: Undergraduate student (Fall 2020 - Summer 2021, co-advised with Shurui Zhou) currently PhD student at UIUC
- Jin Zhou: Engineering Science student (Fall 2020 - Summer 2021) currently PhD student at Cornell
- Lucy Lu: Engineering Science student (Fall 2020 - Summer 2021) currently MS student at Stanford
- Marko Huang: Engineering Science student (Fall 2020 - Summer 2021) currently MS student at University of Toronto
- Gabriel Deza: Engineering Science student (Fall 2020 - Summer 2021) currently MS student at UC Berkeley
- Tejumade Afonja: Research Intern (Summer 2020) currently PhD student at Saarland University
- Milad Nasr: Google Brain Intern (Summer 2020, co-hosted with Nicholas Carlini) currently Research Scientist at Google Brain
- Lorna Licollari: Research Intern (Summer 2020) currently Engineering Science student at University of Toronto
- Pratyush Maini: Research Intern (Summer 2020) currently PhD student at CMU
- Yunxiang Zhang: Research Intern (Spring 2020) currently PhD student at Chinese University of Hong Kong
- Saina Asani: Research Assistant (Winter 2020 - Summer 2020) currently AI Researcher at Huawei
- Laura Zhukas: Undergraduate Student Researcher (Fall 2019) currently BASc student at the University of Waterloo
- Christopher Choquette-Choo: Engineering Science student (Fall 2019 - Summer 2020) currently Research Engineer at Google Brain
- Baiwu Zhang: MEng student (Fall 2019 - Summer 2020) currently ML Engineer at Twitter
- Varun Chandrasekaran: Visiting PhD student (Fall 2019) currently Assistant Professor at UIUC
- Vinith Suriyakumar: MS student (Fall 2019 - Summer 2021, co-advised with M. Ghassemi and A. Goldenberg) currently PhD student at MIT
- Lucas Bourtoule: MASc student (started Fall 2019) currently Security Engineer at Trail of Bits
- Adelin Travers: PhD student (Fall 2019 - Summer 2021, co-advised with David Lie) currently Senior ML Assurance Engineer at Trail of Bits
- Hadi Abdullah: Google Intern (Summer 2019, co-hosted with Damien Octeau) currently Researcher at Visa Research
- Matthew Jagielski: Google Brain intern (Summer 2019) currently Research Scientist at Google Brain
Information for prospective graduate students and postdocs
- If you are interested in joining my research group as a graduate student, apply to the CS or ECE (select "software systems" field) program. Unfortunately, I cannot respond to all prospective graduate students, so the best time is to contact me after you submitted your application.
- If you are interested in joining my research group as a postdoc, please send me an email directly with your CV and research statement.
Research Talks
Upcoming
Here is a list of talks I will be giving. Feel free to reach out if you will be attending one of these events and would like to meet.
Past Recorded Talks
These video resources are a good overview of my research interests.
Blog Posts
Here is a list of blog posts discussing some of the research questions I'm interested in:
- How to prompt LLMs with private data?
- We need a 21st century framework for 21st century problems
- Can stochastic pre-processing defenses protect your models?
- Are adversarial examples against proof-of-learning adversarial?
- How to Keep a Model Stealing Adversary Busy?
- All You Need Is Matplotlib
- How to deploy machine learning with differential privacy? (DifferentialPrivacy.org)
- Arbitrating the integrity of stochastic gradient descent with proof-of-learning
- Beyond federation: collaborating in ML with confidentiality and privacy
- Is this model mine?
- Why we should regulate information about persons, not personal information
- To guarantee privacy, focus on the algorithms, not the data
- Teaching Machines to Unlearn
- In Model Extraction, Don’t Just Ask How?: Ask Why?
- How to steal modern NLP systems with gibberish?
- The academic job search for computer scientists in 10 questions
- How to know when machine learning does not know
- Machine Learning with Differential Privacy in TensorFlow
- Privacy and machine learning: two unexpected allies?
- The challenge of verification and testing of machine learning
- Is attacking machine learning easier than defending it?
- Breaking things is easy
Teaching
- [Fall 2023] ECE421H: Introduction to Machine Learning (see Quercus for course details)
- [Fall 2022] ECE1784H/CSC2559H: Trustworthy Machine Learning
- [Fall 2022] ECE421H: Introduction to Machine Learning (see Quercus for course details)
- [Fall 2021] ECE1784H/CSC2559H: Trustworthy Machine Learning
- [Fall 2021] ECE421H: Introduction to Machine Learning (see Quercus for course details)
- [Fall 2020] ECE421H: Introduction to Machine Learning (see Quercus for course details)
- [Fall 2020] ECE1513H: Introduction to Machine Learning (see Quercus for course details)
- [Winter 2020] ECE1513H: Introduction to Machine Learning (see Quercus for course details)
- [Fall 2019] ECE1784H: Trustworthy Machine Learning